Artificial intelligence (AI) is an active field of research and development with numerous applications. Generative AI, a newer technique, focuses on creating content—learning from large datasets to generate new text, images and other outputs. In 2024, many healthcare organizations embrace generative AI, particularly in creating chatbots. Chatbots, which facilitate human-computer interactions, have existed for a while, but generative AI now enables more natural, conversational exchanges, closely mimicking human interactions. Generative AI is not a short-term investment or a passing trend, this is a decade-long effort that will continue to evolve as more organizations adopt it.
Leveraging Generative AI
When implementing generative AI, healthcare organizations should consider areas to invest in, such as employee productivity or supporting healthcare providers in patient care.
Key factors to consider when leveraging generative AI:
- Use case identification: Identify a challenge that generative AI can solve, but do not assume it will address all problems. Evaluate varying levels of burden reduction across use cases to determine its value.
- Data: Ensure enough data is available for generative AI to provide better services. Identify inefficiencies in manual tasks and ensure data compliance, as AI results depend on learning from data.
- Responsible AI: Verify that the solution follows responsible AI guidelines and Federal recommendations. Focus on accuracy, addressing hallucinations where incorrect information is provided such as responses that are grammatically correct but do not make sense or are outdated.
- Total cost of ownership: Generative AI is expensive, especially regarding hardware consumption. Consider if the same problem can be solved with more optimized models, reducing the need for costly hardware.
Harnessing LLMs for Healthcare
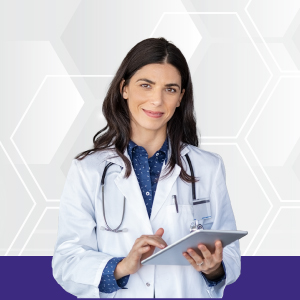
Natural language processing (NLP) has advanced significantly in recent decades, heavily relying on AI to process language. Machine learning, a core concept of AI, enables computers to learn from data using algorithms and draw independent conclusions. Large language models (LLMs) combine NLP, generative AI and machine learning to generate text from vast language datasets. LLMs support various areas in healthcare, including operational efficiency, patient care, clinical decision support and patient engagement post-discharge. AI is particularly helpful in processing large amounts of structured and unstructured data, which often goes unused.
When implementing AI in healthcare, responsible AI and data compliance are crucial. Robustness refers to how well models handle common errors like typos in healthcare documentation, ensuring they can accurately interpret how providers write and speak.
Fairness, especially in addressing biases related to age, origin or ethnicity, is also critical. Any AI model must avoid discrimination; for instance, if a model’s accuracy for female patients is lower than for males, the bias must be addressed. Coverage ensures the model understands key concepts even when phrasing changes.
Data leakage is another concern. If training data is poorly partitioned, it can lead to overfitting, where the model “learns” answers instead of predicting outcomes from historical data. Leakage can also expose personal information during training, raising privacy issues.
LLMs are often expensive, but healthcare-specific models outperform general-purpose ones in efficiency and optimization. For example, healthcare-specific models have shown better results than GPT-3.5 and GPT-4 in tasks like ICD-10 extraction and de-identification. Each model offers different accuracy and performance depending on the use case. Organizations must decide whether a pre-trained model or one trained using zero-shot learning is more suitable.
Buy Versus Build
When it comes to the “buy versus build” decision, the advantage of buying is the decreased time to production compared to building from scratch. Leveraging a task-specific medical LLM that a provider has already developed costs a healthcare organization about 10 times less than building their solution. While some staff will still be needed for DevOps to manage, maintain and deploy the infrastructure, overall staffing requirements are much lower than if building from the ground up.
Even after launching, staffing requirements are not expected to decrease. LLMs continuously evolve, requiring updates and feature enhancements. While in production, software maintenance and support costs are significantly lower—about 20 times less—than trying to train and maintain a model independently. Many organizations that build their healthcare model quickly realize training is extremely costly in terms of hardware, software and staffing.
Optimizing the Future of Healthcare
When deciding on healthcare AI solutions, especially with the rise of generative AI, every healthcare organization should assess where to begin by identifying their pain points. They must ensure they have the data required to train AI models to provide accurate insights. Healthcare AI is not just about choosing software solutions; it is about considering the total cost of ownership for both software and hardware. While hardware costs are expected to decrease, running LLMs remains a costly endeavor. If organizations can use more optimized machine learning models for specific healthcare purposes instead of LLMs, it is worth considering from a cost perspective.
Learn how to implement secure, efficient and compliant AI solutions while reducing costs and improving accuracy in healthcare applications in John Snow Labs’ webinar “De-clutter the World of Generative AI in Healthcare.”
Discover how John Snow Labs’ Medical Chatbot can transform healthcare by providing real-time, accurate and compliant information to improve patient care and streamline operations.