Smaller state agencies need out-of-the-box options that solve immediate needs without a lot of funding or skilled machine learning expertise. Combining RAG with pre-trained LLMs and the agency’s own data accelerates development of AI capabilities and speeds time to value.
In my role at HPE over the last two years, I’ve had meetings with government agencies, defense departments, and research institutions around the world about AI. We’ve discussed everything from how to identify the right use cases for AI, to ethical concerns to getting a handle on the wild, wild west of AI projects across their organizations.
Some of these larger public sector organizations and government agencies have received funding from sources like the U.S. National Science Foundation, U.S. Defense Advanced Research Projects Agency (DARPA), the European Commission’s EuroHPC Joint Undertaking (EuroHPC JU), or the European Defense Fund, which has allowed them to develop AI centers of excellence and build end-to-end AI solutions. They have far-reaching goals — goals such as building the first large language model (LLM) for their native language, becoming the first sovereign, stable, secure AI service provider in their region, building the world’s most sustainable AI supercomputer, or becoming the world leader for trustworthy and responsible AI.
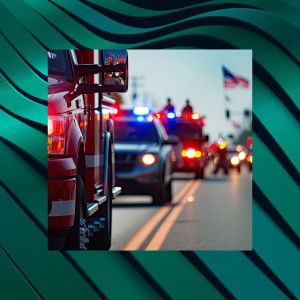
But it takes a lot of resources to train an AI model. The infrastructure needed to train a foundational model may include thousands of GPU-accelerated nodes in high performance clusters. Data scientists and machine learning (ML) engineers are also needed to source and prepare datasets, execute training, and manage deployment.
That’s why many agencies are looking for out-of-the-box options that bring rapid capabilities for solving immediate challenges. Many of these are state and local agencies and higher education institutions. They don’t have the same level of requirements, funding, or expertise to build their own LLMs.
So does that mean the door to powerful AI models is closed on smaller state and local agencies?
No — not if you can gain an understanding of the available pre-trained models that can generate value with AI immediately. There is so much that can be accomplished without ever training a model yourself.
Inference is AI in Action
What exactly is inference? It’s the use of a previously trained AI model such as an LLM to make predictions or decisions based on new, previously unseen data.
Sound complicated? It’s just a fancy way of saying that you’re using an existing model to generate outputs.
In contrast with model training, which involves learning from a dataset to create the model, inference is using that model in a real-world application. Inferencing with pre-trained models reduces both funding requirements as well as the amount of expertise needed to deploy and monitor these models in production.
The pre-trained model market has been steadily growing, as have the number of cloud, SaaS, and open source inference options available. Open AI’s GPT-4o, Anthropic’s Claude, Google’s Gemini, and Mistral AI are among the most popular LLMs used for text and image generation. They’re just some of the thousands of models available through libraries like NVIDIA NGC and HuggingFace.
And just last month in Las Vegas, HPE also made an important announcement of their new NVIDIA AI Computing by HPE portfolio of co-developed solutions. These solutions include HPE’s Machine Learning Inference Software (MLIS), which makes it easy to deploy pre-trained models anywhere including inside your firewall.
Pre-trained Models with Your Data
The advantages of running a pre-trained model with the right platform seem pretty clear — you get the capabilities without the costs of training. However, it’s important to note that a pre-trained LLM excels in general language understanding and generation but is trained on some data other than your own. This is great for use cases where broad knowledge is sufficient and the ability to generate coherent, contextually appropriate text are essential.
So what do you do if you need to generate more specific and up-to-date outputs? There is another machine learning (ML) technique called retrieval augmented generation (RAG) which combines the pre-trained LLM with an additional data source (such as your own knowledge base). RAG combines LLM capabilities with a real-time search or retrieval of relevant documents from your source. The resulting system works like an LLM that’s been trained on your data, but with even more accuracy. RAG is particularly useful for tasks requiring specific domain knowledge or recent data.
Improving Outcomes for State Agencies
Getting started with AI models begins with understanding which problem you want to solve and whether it is most efficiently and effectively solved with AI. Here are some ways different kinds of organizations can leverage pre-trained LLMs:
Law enforcement agencies can use pre-trained models for incident reporting and documentation, to analyze crime data for predictive policing, or to analyze audio and video transcription for evidence management. They can improve community engagement through sentiment analysis and reduce administrative burdens through automated report generation.
Conversational AI can also make many types of citizen services more efficient and user-friendly — from permit applications to public query engines for local government agencies. And LLMs can automate document processing, reducing manual tasks for government workers and improving speed and accessibility of services to citizens.
LLMs can enhance the education experience for students and reduce the burden on teachers. AI-powered virtual assistants can provide tutoring and study support to students outside of school hours and assist researchers in conducting literature reviews by summarizing academic papers or extracting information.
As you consider leveraging pre-trained LLMs, think about the unique problems your agency or institution faces and how this approach could quickly solve those challenges without the need for extensive expertise or the burden and cost of training a model from scratch.
Final Thoughts
As the world and society evolves, the relationship between citizens and their governments, students and their teachers, will evolve too. In fact, they already are. Taking advantage of pre-trained models to solve long-standing automation issues or cumbersome documentation processes can give your organization the catalyst it needs to modernize to meet these new dynamics.
AI is being democratized by a growing number of pre-trained LLMs that are available off the shelf. And you don’t need to have complex data science skills to leverage them, just the right tools.
The door to AI is open for state and local agencies, regardless of size or sophistication. A part of my job is to understand the challenges and goals of public sector organizations of all sizes when it comes to AI.
To learn more about HPE Private Cloud AI, visit the Private Cloud AI solutions overview page and contact the HPE team for questions and comments.
This post originally appeared on HPE.com and is re-published with permission.